Complete the form to view the episode
In this episode, Kabir Patel and Shashank Garg explore the emergence of Agentic AI—intelligent systems capable of autonomous decision-making—and its transformative impact on the pharmaceutical industry. Kabir highlights how AI is evolving beyond automation, enabling autonomous decision-making and breakthrough innovations in drug discovery and materials science. They also explore how AI is shifting from standardization to hyper-personalization, making tailored experiences more accessible. With enterprises at a turning point, the discussion delves into how AI is redefining work, reshaping industries, and unlocking new possibilities.
Complete the form to view the episode
In this episode, Prateek Shrivastava, Advanced Analytics Manager at Cummins and Shashank Garg discuss the transformative impact of AI and data science on supply chains. They discuss how businesses are using AI to proactively address challenges, from predicting truck failures to preventing inventory shortages. Prateek also explores the shift from traditional BI to AI-driven insights and how smart tools are strengthening Cummins’ supply chain resilience. Tune in for an insightful conversation on how technology is reshaping the future of supply chain management!
Complete the form to view the episode
Suvajit Basu, Entrepreneur, CIO, and IT leader at top CPG firms, joins Shashank Garg for an insightful conversation on how Generative AI is shaking up Business Intelligence. They dive into how AI is pushing past traditional static dashboards and transforming decision-making with real-time, dynamic insights. Suvajit shares why solid data foundations are crucial and why businesses need to embrace an AI-first mindset to stay ahead. It’s not just about replacing BI – it’s about rethinking how AI and BI can work together to unlock smarter, faster, and more impactful decisions.
You might also like
You might also like
You might also like
Artificial intelligence (AI) has become increasingly integral to the way we live our lives, providing innovative solutions to complex challenges and transforming various sectors. However, the rapid growth of AI also raises concerns around issues such as data privacy, regulatory compliance, and the ethical use of data. As a result, having a responsible AI framework in place is vital for organizations to ensure trustworthiness and transparency in their AI systems.
In this blog, we will delve into two critical aspects of the compliance component of a responsible AI framework:
- Ensuring data is acquired fairly, with consent, and in compliance with privacy laws.
- Ensuring regulatory and privacy law compliance for users affected by AI recommendations.
Ensuring Fair Data Acquisition with Consent, and Compliance
- Fair Data Acquisition
The foundation of robust AI solutions is the quality and the method of acquiring the data used for the training and validation of algorithms. Ensuring fair data acquisition means collecting data by adhering to principles that prevent discrimination, promote inclusiveness, and consider user consent.
- The Role of Data Diversity
Creating inclusive AI models starts with gathering diverse data sets that represent different demographic groups, regions, and contexts. Ensuring this diversity helps prevent algorithms from favoring any particular group and maintains fairness across the AI system.
- Mitigating Bias
Since AI models depend on the quality and characteristics of the input data, they can inherit biases present in the data. Bias in AI systems may lead to unfair results, reinforcing existing stereotypes or discriminating against certain populations. Organizations should take active steps to identify, assess, and mitigate potential biases in the data collection process.
- Data Acquisition with Consent
Consent is a vital aspect of acquiring data fairly. Users must be both informed about and explicitly agree to their data’s collection, use, and storage. Consent must be specific, freely given, and easily revocable by the data subject.
- Privacy-By-Design Approach
Taking a privacy-by-design approach means considering privacy and data protection throughout the entire data lifecycle, from collection to disposal. This approach allows organizations to incorporate privacy measures directly into AI system designs, ensuring compliance with data protection regulations.
- Compliance with Privacy Laws
AI development has led to an increased emphasis on data privacy laws around the world. As a result, organizations must ensure that data acquisition practices align with applicable privacy regulations, such as GDPR in Europe or CCPA in California. Compliance necessitates transparency with users, obtaining appropriate consent, and only using data within the terms of these agreements.
Regulatory and Privacy Law Compliance for Users Affected by AI Recommendations
The impact of AI technologies on everyday life can be profound. As AI-driven tools increasingly provide recommendations affecting people’s jobs, healthcare, and more, ensuring regulatory and privacy law compliance becomes especially crucial.
- Monitoring and Evaluation
Constant monitoring and evaluation of AI systems can help organizations identify potential biases, ensure the accuracy of AI recommendations, and comply with regulations. Methods such as auditing models, reviewing inputs, and analyzing outputs can enable businesses to detect and correct any AI recommendation that does not align with compliance and ethical standards.
- Transparency and Explanations
Given that AI systems’ recommendations affect users, it’s essential to make AI algorithms transparent and explainable. Providing users with clear reasons behind AI recommendations helps promote trust in the technology and allows users to understand the data processing and factors considered when reaching a conclusion.
- Data Protection and Privacy of Affected Users
The protection of users’ privacy and personal data is a cornerstone of regulatory compliance. Implementing strong data protection practices and giving users control over their personal information can help organizations respect user privacy and balance the benefits of AI technology with its potential risks.
- Anonymization Techniques
Effective anonymization techniques can help organizations protect user privacy by stripping data of identifying information, while still using it to inform AI models. Methods such as differential privacy or tokenization can support businesses in maintaining compliance while still benefiting from AI’s potential.
- Legal Compliance in AI-driven Decision-making
AI-driven recommendations may have substantial legal ramifications, particularly in specific sectors like finance, healthcare, and employment. Organizations need central AI governance frameworks to oversee models’ compliance with sector-specific regulations and address potential ethical tensions.
In Summary…
The adoption of AI technologies has the potential to unlock enormous societal and economic benefits. However, to maximize these benefits and minimize risks, businesses must work tirelessly to ensure that their AI systems are developed and deployed responsibly.
The compliance component of a responsible AI framework focuses on fair data acquisition practices, obtaining consent, and upholding privacy and regulatory standards. By embedding compliance and ethical principles at the core of AI system design, organizations can thrive in the AI landscape, nurture users’ trust, and deliver positive outcomes for all stakeholders.
To explore the other parts in this series, click here.
Recent Blogs
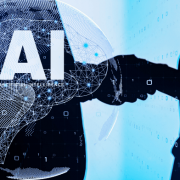
Bots to Brains: How Agentic AI is Changing the Game
July 8, 2025
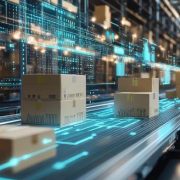
The Future of Supply Chains Is AI-Driven—Is Your Business Ready to Embrace the Change?
July 2, 2025
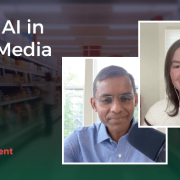
What Retail Media Can Learn from Instacart’s AI Strategy
June 24, 2025
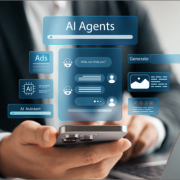
Beyond Chatbots: How Agentic AI Is Automating High-Stakes Business Decisions
June 11, 2025
Reliability is one of the foundations of trust when it comes to effective artificial intelligence (AI) systems. Without it, user trust can be swiftly eroded, bringing into question any beneficial outcomes. Here, we discuss five key facets of reliability within an AI framework:
Monitoring and Alerts in the World of AI
The heartbeat of an AI system, much like in biological creatures, can indicate when things are functioning well, or when conditions might be headed towards critical states. By embedding monitoring protocols into AI systems, we can alert human supervisors when outputs deviate from expected norms. Consider the analogy of a self-driving car equipped with a system that triggers a warning when the vehicle encounters circumstances that deviate from acceptable parameters, such as a sudden change in weather. In an AI context, machine learning models that form the core of many AI applications can deviate from their training when they encounter data significantly different from the data on which they were trained. In this case, monitoring and alert systems could provide early indicators of ‘drift’ in model performance, allowing human supervisors to intervene swiftly when required.
Contingency Planning
Contingency planning is akin to having a well-rehearsed emergency protocol that guides actions when errors occur in the system. Under the hood of many industry-leading AI systems, contingency plans often take the form of fallback procedures or key decision points that can redirect system functionality or hand control back to human operators when necessary. In healthcare AI, for example, contingency planning might involve supplementary diagnostic methods if the AI system registers an unexpected prognostic output. It is critical to pre-empt potential failings of an AI system, charting a path ahead of the time that enables us to respond effectively when the unexpected occurs.
Trust and Assurance
Trust, that ethereal quality, is not a one-time establishment in AI systems but an ongoing, ever-refreshing assurance to users about the system’s reliability. A banking AI application, for example, would be challenged to win over customers if it didn’t consistently meet or exceed their expectations. To establish trust, AI systems should reliably function within their intended parameters. Regular testing and validation of the AI modules can ensure the system’s dependable service and promote users’ confidence. When users witness first-hand the system’s performance and responsiveness to their needs, trust is reinforced. In this delicate arena, transparency about system operations and limitations contributes significantly towards nurturing user trust, maintaining the relationship with the technology and its human benefactors.
Audit Trails
Audit trails are like breadcrumbs, revealing the steps taken by the AI system in reaching a conclusion. They offer transparency and facilitate interpretation, helping users to understand complex decision-making processes. In a legal AI system, for example, providing justifications for case predictions can foster trust by making the technology more approachable. Moreover, audit trails enable accountability, a fundamental principle for responsible AI. They allow us to trace any systemic malfunctioning or erroneous decision-making back to their origins, offering opportunities to rectify faults and prevent recurrence.
Data Quality
Data quality is the compass by which AI systems navigate. Low-quality data can lead our intelligent systems astray, sabotaging their expected performance and reliability. Ensuring data quality involves careful curation, detangling biases, removing errors, and confirming the data’s relevance to the problem at hand. Take environmental AI, for instance, where data such as climate patterns, pollution levels, and energy consumption form inputs to predictive models forecasting weather changes. If the quality of data is poor in any measurement, the forecasts – and so the reliability – of the AI system are at stake. Therefore, consistent checks and validation processes should be conducted to maintain the credibility of the data, underpinning the reliability of the whole system.
In essence, reliability in AI is a holistic exercise underpinned by vigilant monitoring of system performance, meticulous contingency planning, persistent trust building, comprehensive audit trails, and unwavering commitment to data quality. Delivering reliable AI is not the end of a journey, but a constant voyage of discovery, innovation, and improvement. Balancing these five pillars of reliability can indeed be a complex task, yet it is an absolutely vital one where AI’s value proposition is considered. By striving for reliability in AI systems, professionals and enthusiasts alike can contribute to more responsible and impactful AI deployments across numerous sectors, harnessing the transformative potential of AI technology.
To explore the other parts in this series, click here.
Recent Blogs
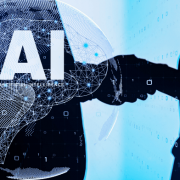
Bots to Brains: How Agentic AI is Changing the Game
July 8, 2025
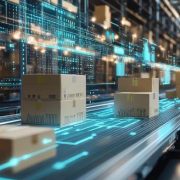
The Future of Supply Chains Is AI-Driven—Is Your Business Ready to Embrace the Change?
July 2, 2025
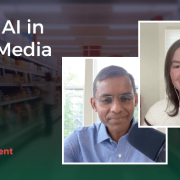
What Retail Media Can Learn from Instacart’s AI Strategy
June 24, 2025
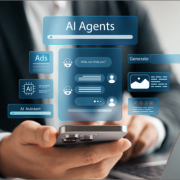
Beyond Chatbots: How Agentic AI Is Automating High-Stakes Business Decisions
June 11, 2025
As technology continually evolves at an impressive rate, artificial intelligence (AI) is becoming an essential part of various industries, including medicine, finance, education, and economics. However, as AI becomes more prevalent, it is absolutely essential that we turn our focus to the security aspect of these systems. The exponential increase in reliance on AI necessitates a framework with unassailable security to safeguard our data and protect our resources.
Importance of Data Security in AI Systems
In the AI realm, data is the backbone of all operations; it fuels the algorithms, drives predictive capabilities, and allows for advanced problem-solving. As the saying goes, “garbage in, garbage out”: without high-quality, accurate data, an AI system is useless at best and dangerous at worst. Therefore, ensuring data security is not just an option or an add-on but a fundamental requirement.
Securing data in AI systems can be challenging because data is continuously flowing – data-in-transit, data-at-rest, and data-in-use, each requiring unique security considerations. Regardless, protecting against cyber threats, leaks, unauthorized access, and tampering should always be prioritized. A breach can not only lead to data loss but also produce incorrect AI outputs, compromising processes and decisions based on those outputs.
Ensuring Access Control and Authentication
The question of ‘who has access’ to data in AI systems is a significant determinant of its overall security posture. Ensuring access control and authentication mechanisms are a part of the integrated security measures in an AI framework.
Having an efficient access control strategy denies unauthorized users access to certain realms of data in the AI system, hence minimizing the risk of a potential data breach. This strategy involves categorizing users and defining their access rights and privileges, giving only the necessary level of access to each category to perform their tasks.
Authentication, on the other hand, is the process of confirming that users are who they claim to be. This process helps keep the AI system secure by preventing fraudulent access or manipulations leading to data breaches. Employing multi-factor authentication (MFA) adds an additional layer of security by requiring users to provide two or more verification factors to gain access.
Security of Data Storage
Last but equally important in the secure AI framework is the security of data storage. Where and how we store our data ultimately determines its security, accessibility, and protection against potential threats.
Data could be stored in one of the three forms, on-premises storage, cloud storage, or hybrid storage. Each of these has its own pros and cons, so an organization must make informed decisions based on their individual requirements and constraints.
Regardless of the storage choice, best practices require data encryption both at rest and during transmission. Encryption renders data unreadable, only allowing access to those possessing a correct encryption key. Regular backups should also be established as a part of a disaster recovery plan.
In addition, it’s crucial to work with trustworthy service providers when using cloud storage solutions. You must ensure adherence to industry-standard protocols and regulatory compliances, such as HIPAA for health information or PCI DSS for credit card data.
Security’s Vital Role in Responsible AI
As we navigate through the intricate world of AI, ensuring the security of our AI systems is paramount. By understanding the importance of data security, implementing robust access control, and placing a high priority on secure data storage, we can greatly mitigate potential security risks.
After all, a responsible AI framework is not only about achieving AI’s full potential. It encompasses gaining the trust in the system’s reliability and accuracy. And without security, there can be no trust. Hence, integrating these components into an AI framework is not just a necessity but an absolute responsibility.
Recent Blogs
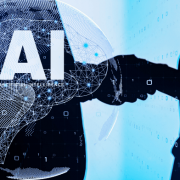
Bots to Brains: How Agentic AI is Changing the Game
July 8, 2025
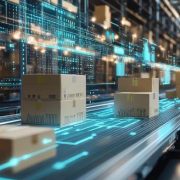
The Future of Supply Chains Is AI-Driven—Is Your Business Ready to Embrace the Change?
July 2, 2025
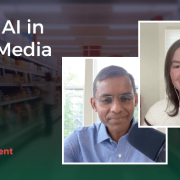
What Retail Media Can Learn from Instacart’s AI Strategy
June 24, 2025
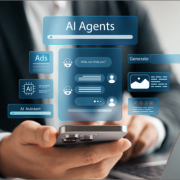
Beyond Chatbots: How Agentic AI Is Automating High-Stakes Business Decisions
June 11, 2025
Explainability is central to the responsible deployment of AI technologies.. It encapsulates the idea that AI systems should not only deliver accurate predictions, but their decision-making processes should be understandable and justifiable for users and stakeholders. Our examination of the topic will include a discussion on how features and data shape AI predictions and explore the significance of human-readable explanations.
Explainability: Building Trust through Understanding
Explainability, at its core, is about making the inner workings of AI systems transparent. It shuns the notion of “black box” AI, which obscures the link between inputs and predictions. This transparency is not merely an academic requirement. It has practical implications in building trust, improving use cases, and complying with regulations that mandate decisions made by AI to be explainable.
“The black box” complexity could potentially lead to unintended and inequitable consequences, particularly in sensitive applications like healthcare, finance, and judiciary systems. With explainability, we introduce accountability, fostering a colocated sense of responsibility and confidence in AI applications.
The Role of Features and Data in AI Predictions
The output of an AI system pivots around the data and features used in its training. Features are variables or attributes chosen as input for the AI model, which based on these, makes predictions. The features chosen and data collected to train the algorithm can significantly impact performance and accuracy.
Consider, for example, an AI system designed to predict patient susceptibility to a particular disease. A well-chosen set of features, such as age, pre-existing conditions, and genetic information, can dramatically influence the prediction accuracy. Similarly, the quality, diversity, and size of the dataset also play an integral part. Faulty, incomplete, or biased data can lead to skewed or unfair predictions.
Human-Readable Explanations: Decoding AI Decision-making
While it is paramount that AI can make accurate predictions, those predictions remain of dubious value if humans can’t interpret them. Human-readable explanations come into play here. Enabling AI to explain its logic in a manner understandable to humans can greatly improve its usability and transparency. Think of it as a translator between the complex mathematical relationships the AI understands and the human language we understand.
Imagine a credit scoring AI that rejects an application. A straightforward “Application denied” message, although accurate, isn’t particularly useful. Instead, a useful response might be: “Your application was denied due to your high debt-to-income ratio and recent default history.” This empowers the applicant with the understanding to improve their credit score.
Explainability is Not an Optional Add-on
The mission of responsible AI frameworks goes beyond accurate predictions. To empower users and build trust in these powerful systems, we must give attention to explainability. Accordingly, carefully choosing features and providing quality data lays the groundwork for predictions made, while fostering an environment where AI can offer human-readable explanation serves as the bridge between machine output and human input.
As we continue to adopt and weave AI even deeper into the fabric of society, it becomes increasingly more critical that we infuse transparency into these systems. Explainability is not an optional add-on, but an essential ingredient for responsible AI, ensuring these powerful tools are accountable, understandable, and ultimately, a force for good.
To explore the other parts in this series, click here.
Recent Blogs
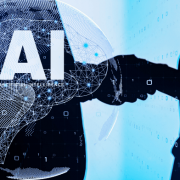
Bots to Brains: How Agentic AI is Changing the Game
July 8, 2025
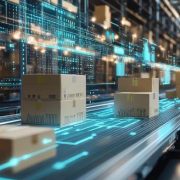
The Future of Supply Chains Is AI-Driven—Is Your Business Ready to Embrace the Change?
July 2, 2025
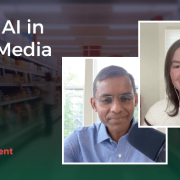
What Retail Media Can Learn from Instacart’s AI Strategy
June 24, 2025
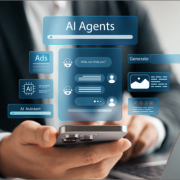
Beyond Chatbots: How Agentic AI Is Automating High-Stakes Business Decisions
June 11, 2025