Data is the most important asset for any organization and is the key to its growth and success. Analytics, which is at the heart of any digital transformation initiative empowers new age organizations to leverage data effectively to make smart decisions.
Every organization today is trying to invest significantly in Analytics and it’s a core area of discussion in board meetings. But then, why do so many Analytics projects fail to make an impact? While there are many reasons for failures, here are the top six that you should be aware of before kicking off any analytics project.
-
Lack of Management Vision & Support:
Top management sets the tone for any organizational initiative and Analytics is no different. Visionary management team will support the need to build a data-driven culture within the organization.
- Humans have the tendency to resist any change. If analytics programs are kicked off just to follow the market trend without setting up the business strategy, it’s bound to fail. Business leaders should include analytics as part their vision and handle the change management effectively through inclusivity and trust building measures.
- Analytics projects are traditionally hard to implement. They typically start with a Proof of Concept(PoC). Bringing analytics projects from conceptualization to production requires clear understanding of business goals to everyone involved in the program. We have seen the crazy race to launch the Analytics programs and many of these projects go down due to lack of management vision and top-down support.
-
Poor or Missing Data:
Data driven originations have survived the most challenging of the conditions because they believed in their data and utilized it to the maximum for accomplishing their executive goals.
- It is important to continuously check the quality of data and make necessary changes to the data cleansing routines as required. Make the Data quality routines flexible so that they can continuously adapt to new rules or sources of data.
- Business processes are becoming more and more complex and end users are continuously looking for new product lines and real time assistance. Understanding the data required for any Analytics model is the key to success.
- Idea is to bring right data by effectively understanding the business requirements and baselining the processes. Data should be able to power analytics engines effectively which can result in actionable insights with greater impact.
- Good data will aid the success of any analytics project and on contrary bad choice or quality of data will make the task difficult and ultimately take down the complete analytics investment.
-
Missing Analytics Governance:
Most of the Analytics projects are built on small PoC/MVPs and once it’s successful, business/product owners demand to build more use cases on top of this without strengthening Analytics Governance processes.
- Analytics governance should go hand in hand with the first ever Analytics project and should not be left aside for future enhancements.
- Things like Security should be the day 0 priority and should not be ignored even when you are delivering a small PoC. Go for an effective role and access management so that the trust is not compromised at any level.
- Analytics Governance ignorance can result in multiple points of failures such as security breaches or reduced performance which eventually may bring the bad name to the organization and complete analytics initiative may go for a toss.
-
More Time to Market:
It is a furious competition out there and if you are not able to deliver on time, someone else will.
- We have seen cases where Analytics programs were delivered but it failed to catch up with the consumers as the competitor was ahead in the game.
- Time to Market from ideation to finished analytics should be a short cycle and idea should be to deliver results quickly to the business through short MVPs.
- From technology point of view use the power of cloud to eliminate infrastructure bottlenecks. This will not only shorten the complete cycle, but you will be able to come back to the drawing board quickly in case of any inadequacies. Adaptation is the key to success and the feedback loop should be kept open.
- Create a lean Project management schedule even if it is a PoC. This not only helps in tracking activities closely, but any bottlenecks can also be managed immediately.
- Have simple visualizations on top of your analytics output which can be easily understood by the business teams. Many of the times we have seen that end users who consume analytic results fail to interpret analytics output and ultimately the UAT cycle is stretched.
-
Missing Appropriate Team members:
While it is okay to have cross trained team members in your analytics team, at least have one strong resource who understands the technology and is curious about what that data might reveal to solve the business question that analytics is trying to answer and bring required Data Science & Machine Learning leadership.
- Working on an Analytics project without business stakeholder’s active engagement can lead to a weak hypothesis and ultimately the Time to market is stretched.
- Keep business stakeholders in your team as product owners from day 1 even for a small PoC. They can be owners of the business process that you are trying to simplify. Having them in your team can reduce the chance of failure as they can continuously provide the feedback to improve your model.
-
Ignoring Regulatory compliance requirements:
Businesses may have to pay heavily if regulatory compliance are missed.
- While analytics for an organization is rewarding, it may become a high-risk issue if it is not organized and compliant to regulatory laws.
- Most of the time Analytics projects are kicked off in a rush and delivered technically without considering the regulatory and compliance requirements for that region/business. For example, if you are working for a European client, GDPR is something which you cannot ignore else you will never be able to productionize your model even if it’s technically super.
Get in touch to know more!
Recent Blogs
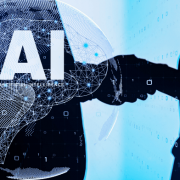
Bots to Brains: How Agentic AI is Changing the Game
July 8, 2025
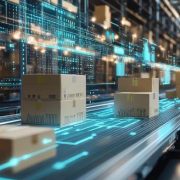
The Future of Supply Chains Is AI-Driven—Is Your Business Ready to Embrace the Change?
July 2, 2025
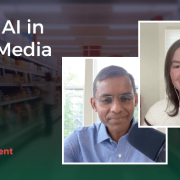
What Retail Media Can Learn from Instacart’s AI Strategy
June 24, 2025
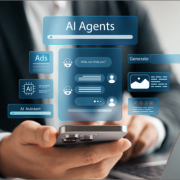
Beyond Chatbots: How Agentic AI Is Automating High-Stakes Business Decisions
June 11, 2025